Core Technologies of Markerless Navigation
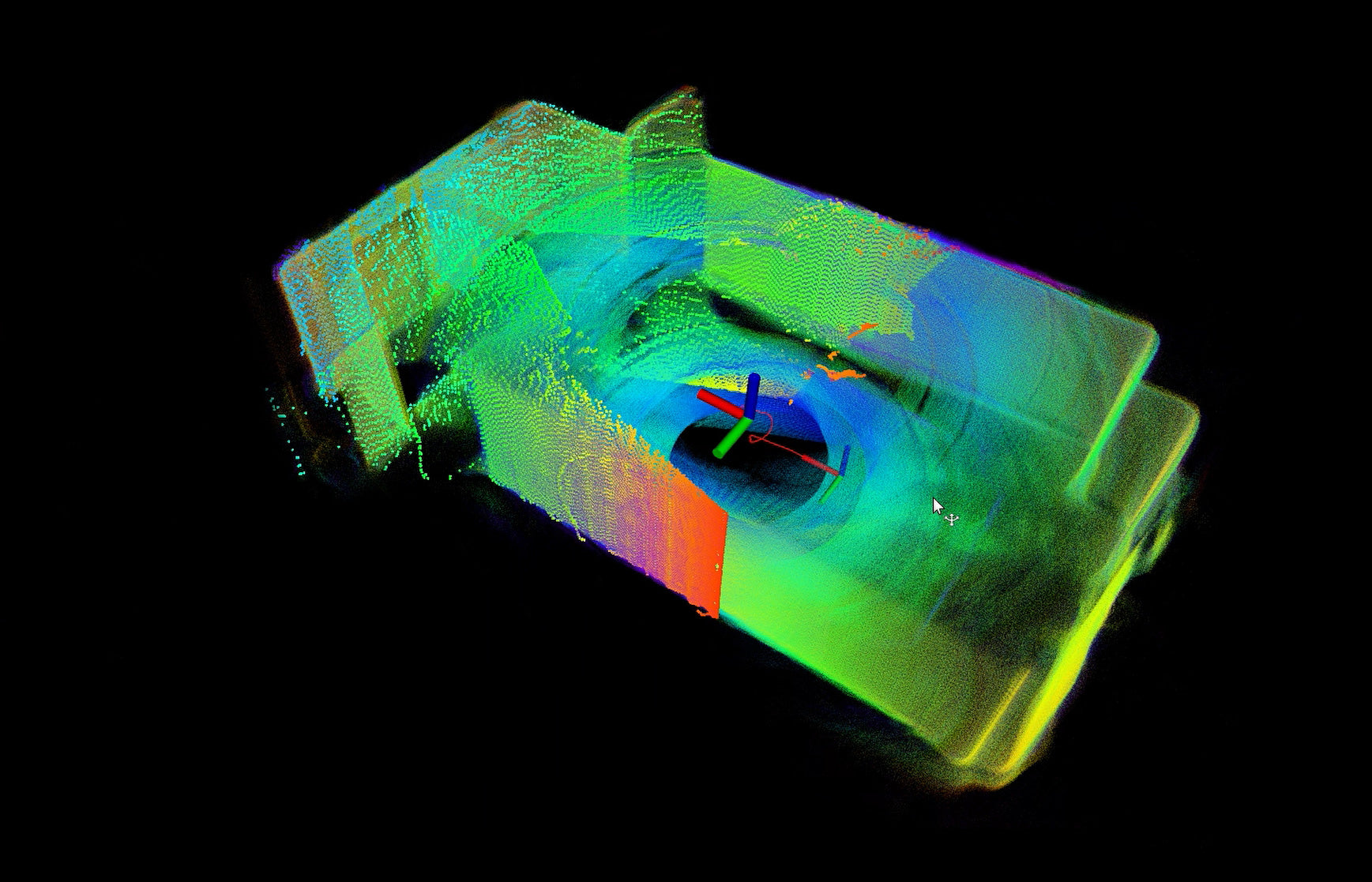
How is markerless navigation achieved? It relies on a series of innovative technologies. Let’s break it down into the following key aspects:
High-Precision Localization and Navigation
The core of markerless navigation is that robots can achieve high-precision localization without relying on any external markers, using SLAM (Simultaneous Localization and Mapping) technology. SLAM allows robots to build environmental maps in real-time and localize themselves, without the need for pre-set maps or markers.
Efficient Path Planning and Decision-Making
In addition to accurate localization, robots need to be able to plan paths efficiently, avoid obstacles, and respond to dynamic changes in the environment. Efficient path planning algorithms like the A algorithm* and deep reinforcement learning help robots adjust their paths in real-time, addressing challenges in complex environments.
Dynamic Obstacle Avoidance and Environmental Interaction
Markerless navigation requires robots to have dynamic obstacle avoidance capabilities. Using LiDAR, cameras, and other sensors, robots can identify and avoid obstacles in the environment. Moreover, they can interact with their surroundings to perform tasks, such as transporting items or avoiding pedestrians.
Comprehensive Environmental Perception and Semantic Understanding
In addition to physical obstacles, robots also need to understand semantic information in their environment. For instance, recognizing and interpreting road signs, furniture layouts, and other contextual clues helps robots make more intelligent decisions.
3. Practical Applications of Markerless Navigation
Next, let’s explore some well-known mobile robots that have already implemented markerless navigation and their real-world applications. This will help to better understand the practical value of the underlying technologies.
(1) Robotic Vacuum: iRobot Roomba
iRobot's Roomba is one of the most famous robotic vacuums, using markerless navigation to efficiently clean floors by detecting obstacles and mapping out the environment.
(2) Autonomous Delivery Vehicles: Nuro
Nuro’s autonomous vehicles are designed for last-mile deliveries, utilizing markerless navigation for precise navigation through urban environments, avoiding pedestrians, and delivering goods without relying on external markers.
(3) All-Terrain Mobile Robots: Clearpath Robotics Husky
Clearpath’s Husky is an all-terrain robot used in research and industrial applications, relying on markerless navigation to navigate outdoor environments, perform tasks, and adapt to changes in terrain.
(4) Intelligent Logistics Robots: Starship Technologies
Starship Technologies’ robots use markerless navigation for efficient parcel delivery in urban settings, avoiding obstacles, interacting with the environment, and operating autonomously.
4. Future Developments in Markerless Navigation
As markerless navigation technology continues to mature, its applications will expand to more fields. Here are some key trends to watch:
- Expanding from Indoor to Outdoor Applications: Markerless navigation has already moved beyond indoor robotic vacuum cleaners to outdoor applications like autonomous driving and logistics delivery. Robots will navigate autonomously in more complex and dynamic environments.
- Multi-Robot Collaboration: In the future, multiple robots will work together using distributed path planning and collaborative algorithms to complete more complex tasks. This requires markerless navigation to not only consider the task of a single robot but also ensure efficient collaboration between robots in shared environments.
- Intelligent Decision-Making and Adaptive Learning: With the advancement of reinforcement learning and deep learning technologies, robots will gain the ability to self-learn and optimize their performance, continuously adapting to changing environments and improving task efficiency.
Markerless navigation enables robots to operate efficiently in complex, dynamic environments without relying on fixed markers. As SLAM technology, multi-sensor fusion, and deep learning continue to evolve, markerless navigation will play an increasingly important role in fields like autonomous driving, logistics delivery, and smart homes.