From High-Precision Maps to Mapless Navigation: The Future of Autonomous Robotics
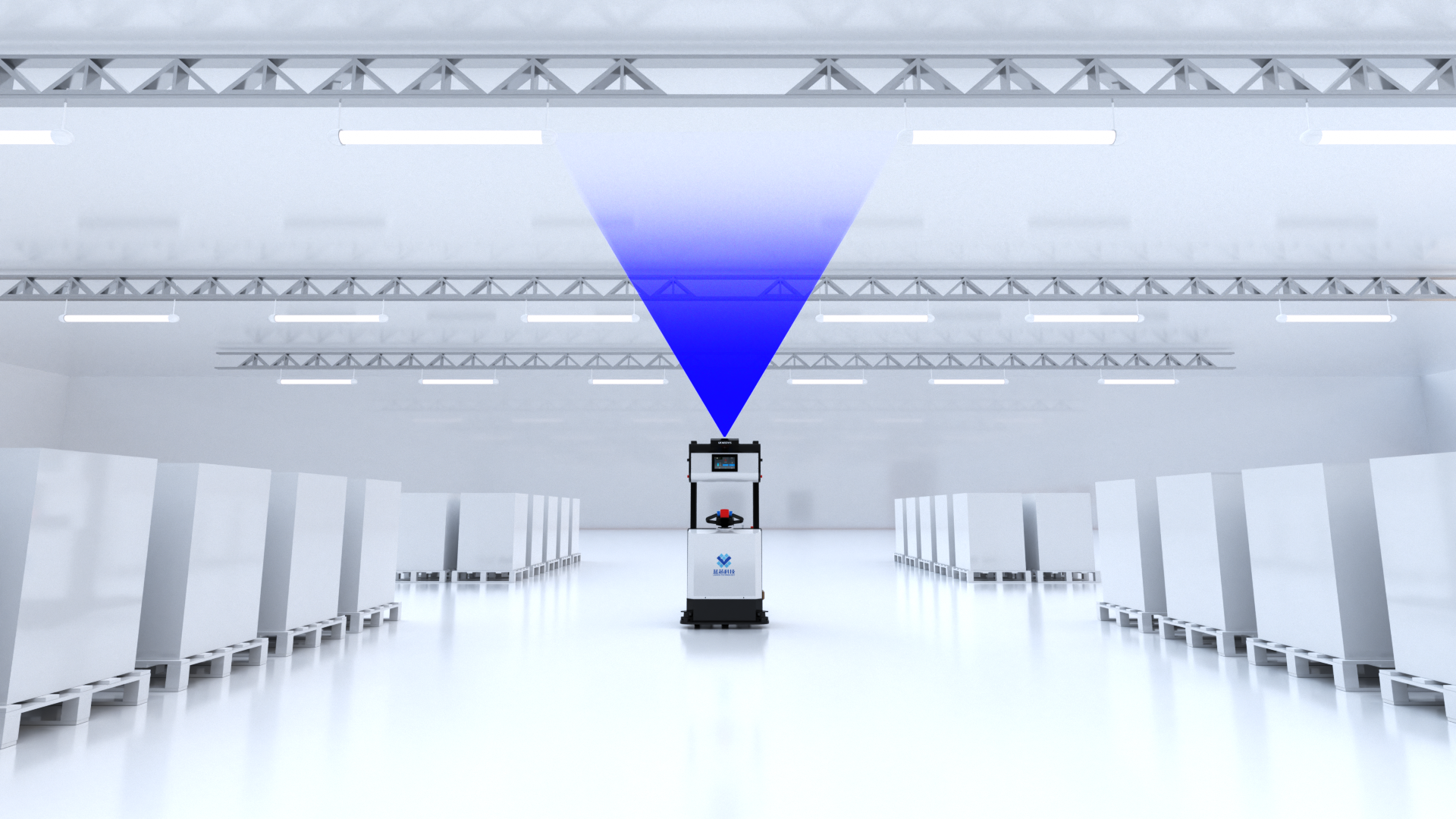
In the early days of robotics, particularly in autonomous driving and warehouse logistics, robots relied heavily on high-precision maps for localization and navigation. These maps, created using LiDAR, high-definition cameras, and other sensors, captured environmental details such as walls, obstacles, and roads.
However, while high-precision maps performed well in known environments, they had significant limitations:
- High costs of map creation and updates;
- Limited adaptability to environmental changes (e.g., temporary obstacles, surface variations, lighting changes);
- Inability to respond in dynamic environments, where unexpected events occur in real time.
As technology has progressed, robots have gradually evolved toward mapless navigation. This approach eliminates the dependency on pre-set maps, allowing robots to navigate autonomously by perceiving the environment in real-time and building dynamic maps as they move.
2. Innovations Required for Mapless Navigation
Achieving mapless navigation requires a combination of advanced technologies that allow robots to navigate without pre-existing maps and rely solely on real-time data to make autonomous navigation decisions.
(1) Real-Time Environmental Perception and Adaptive Localization
- Sensor Technologies: Mapless navigation demands that robots possess robust environmental awareness. They typically use a combination of LiDAR, RGB-D cameras, ultrasonic sensors, and IMUs (Inertial Measurement Units) to gather real-time data and build dynamic maps.
- SLAM Technology: With visual SLAM or LiDAR SLAM, robots can localize themselves and build maps in real-time in both unknown and dynamic environments. SLAM technology allows robots to update maps dynamically, helping them adjust to environmental changes and maintain accurate positioning.
- Adaptive Localization: Robots must be capable of self-adjusting their position and path when environmental changes occur, such as moving obstacles or changes in the terrain. Mapless navigation completely relies on sensor input for autonomous localization, without the need for external markers.
(2) Dynamic Path Planning and Real-Time Decision-Making
- Real-Time Path Planning: Efficient path planning is essential for mapless navigation, especially when robots are operating at high speeds. Robots generate optimal paths in real-time using the sensory data, avoiding obstacles and adapting to sudden changes in the environment.
- Deep Learning and Intelligent Decision-Making: Real-time decision-making is critical in mapless navigation. These decisions are based on environmental perceptions and historical data, allowing the robot to make intelligent adjustments as the environment evolves.
- Dynamic Path Optimization: Robots operating without maps can dynamically optimize their paths when encountering obstacles or changes in the environment. With technologies such as deep reinforcement learning, robots can continuously improve their navigation strategies through interaction with the environment.
(3) Multi-Sensor Fusion and Environmental Understanding
- Multi-Sensor Fusion: In mapless navigation, robots combine inputs from various sensors like vision, LiDAR, and ultrasonic sensors to enhance navigational accuracy and stability. By fusing data from these sensors, robots can understand and react to their environment in real-time.
- Semantic Understanding of the Environment: Beyond detecting physical obstacles, robots must also possess semantic understanding. Through deep learning, robots can identify and interpret objects like people, vehicles, road signs, and more. This understanding enables robots to make decisions based on the context of these objects, such as differentiating between pedestrians and stationary obstacles and responding accordingly.
(4) Efficient Computing and Low-Latency Data Processing
- Edge Computing: To process the vast amounts of sensor data in real time, robots often use edge computing, where data is processed locally rather than being uploaded to the cloud. This reduces data transmission latency and ensures quick responses to environmental changes.
- Low-Latency Communication: In the case of multi-robot collaboration, low-latency communication protocols (such as 5G or Wi-Fi 6) are essential to enable real-time data exchange and decision-making.
3. Practical Applications of Mapless Navigation
Let’s explore several mobile robots that have already successfully implemented mapless navigation technologies, demonstrating the practical applications and potential of this innovation.
(1) Autonomous Vehicles
-
Representative Products: Waymo, Tesla Autopilot
- Technology: These autonomous driving systems do not rely on high-precision maps. Instead, they use visual SLAM, LiDAR, and camera sensors to perceive the environment in real-time and navigate without the need for preset maps.
- Use Case: Autonomous vehicles can achieve mapless navigation on city streets and highways, avoiding obstacles and making real-time decisions on the road.
(2) Delivery Robots: Nuro
-
Representative Product: Nuro Autonomous Delivery Vehicle
- Technology: Nuro uses LiDAR and visual sensors for mapless navigation, enabling it to operate in complex urban environments.
- Use Case: This robot is already being used for urban delivery, autonomously avoiding pedestrians, vehicles, and other dynamic obstacles while delivering goods.
(3) All-Terrain Mobile Robots: Clearpath Robotics Husky
-
Representative Product: Husky Robot
- Technology: Husky uses visual SLAM and LiDAR technology for all-terrain autonomous navigation without relying on high-precision maps. It is widely used in research, logistics, and agriculture.
- Use Case: With mapless navigation, Husky can traverse uneven or irregular terrain, providing stable navigation even in challenging environments.
The evolution from high-precision maps to mapless navigation marks a significant leap in robotic mobility. Mapless navigation frees robots from dependence on fixed maps and markers, allowing them to operate in more complex, dynamic environments while adapting to environmental changes.
With the ongoing advancements in SLAM technology, multi-sensor fusion, and deep learning, future robots will demonstrate greater autonomy and flexibility across a variety of industries, including autonomous driving, warehouse logistics, and agriculture. The breakthrough of mapless navigation will enable robots to transition from constrained environments to open, dynamic environments, broadening their applicability and potential.